Salesforce has claimed that its new slimline “Tiny Giant” LLM outperforms larger models and potentially marks a leap forward in the development of on-device AI.
In a paper published on Arxiv, Salesforce’s AI Research department reported that its xLAM-7B LLM model came in sixth place among 46 models including AIs from OpenAI and Google in a competition testing function-calling (the execution of tasks or functions through API calls).
This plucky, parsimonious LLM was trained on just seven billion parameters - a fraction of the 1.7 trillion parameters rumoured to have been used to educate GPT 4 (although OpenAI has not confirmed this figure).
However, Salesforce sees its true star as the Tiny Giant - xLAM-1B - which finished in 24th place - which Salesforce described as an “exceptional performance, surpassing GPT-3.5-Turbo and Claude-3 Haiku.”
This showing was impressive because the 1B in the model's not-so-snappy name indicates it was trained on just one billion parameters.
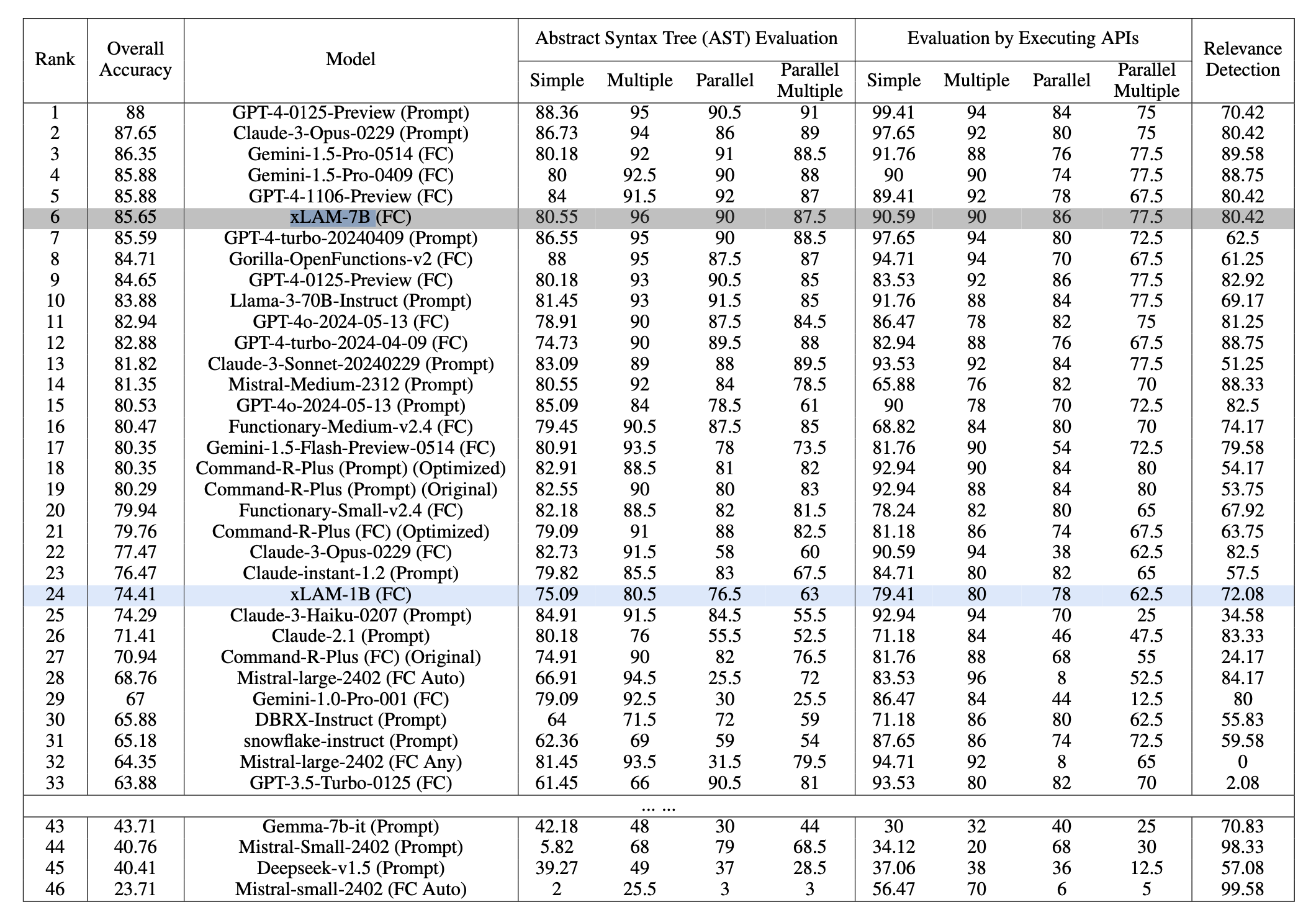
The news prompted Marc Benioff, Salesforce chair and CEO, to make the bold claim that this diminutive LLM is now the world’s top-performing “micro-model” for function-calling.
Benioff shared the results of this research in a post on X which said: “Meet Salesforce Einstein ‘Tiny Giant.’ Our 1B parameter model xLAM-1B is now the best micro model for function calling, outperforming models 7x its size… On-device agentic AI is here. Congrats Salesforce Research!”
The Salesforce paper said that function-calling agents “represent a significant advancement” in AI and, specifically, LLMs.
Models such as GPT4, Gemini, and Mistral already execute API calls based on natural language prompts. This could involve, for instance, asking the model about the weather and then having the data pulled and presented immediately from a forecaster’s API.
“This capability extends the utility of LLMs beyond simple conversation tasks to include dynamic interactions with a variety of digital services and applications, ranging from social media platforms to financial services,” Salesforce wrote.
However, many of the most popular models are large, unwieldy and resource-intensive, requiring access to the computational power of cloud data centres and external infrastructure.
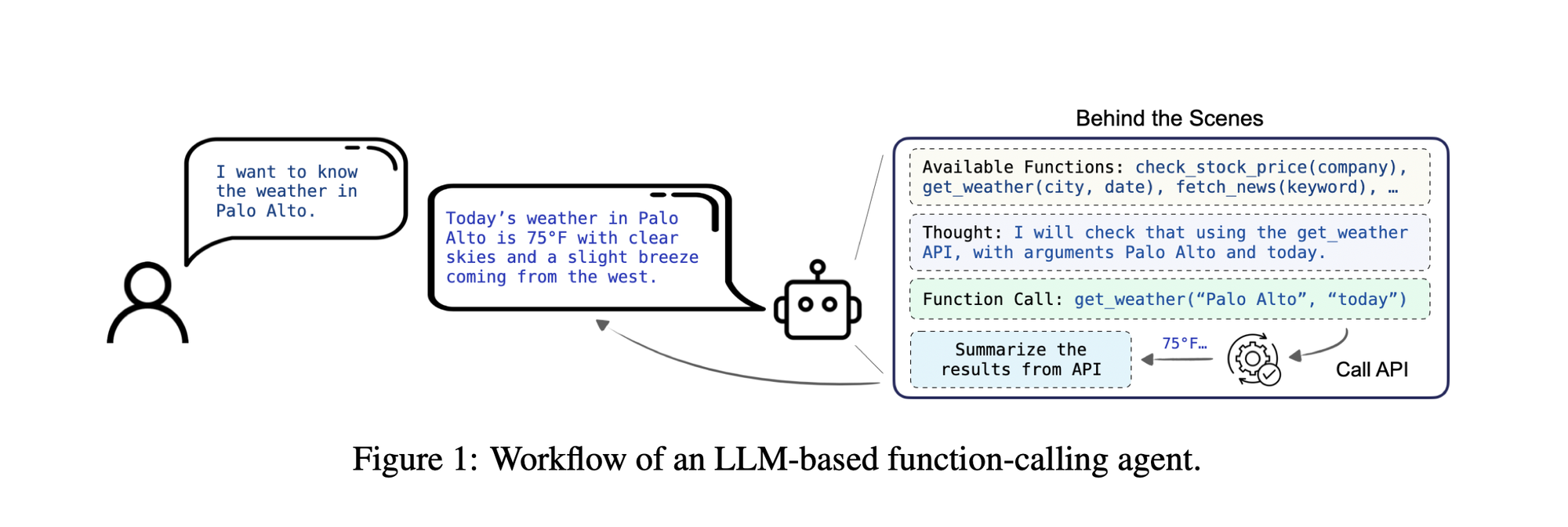
To test the performance of function-calling LLMs, Salesforce built APIGen, an “Automated Pipeline for Generating verifiable and diverse function-calling datasets”, which synthesises data for AI training.
“Our framework is designed to facilitate the fine-tuning of function-calling LLMs by providing high-quality, diverse datasets that better reflect the variability and complexity of real-world API use,” it wrote.
Its critical takeaway was that models trained on relatively small datasets can outperform those fed much larger amounts of information.
“Models trained with our curated datasets, even with only seven billion parameters, can achieve state-of-the-art performance… outperforming multiple GPT-4 models,” Salesforce wrote.
Ultimately, the dream is to create agentic AI models that can carry out function calling and task execution on devices, providing operations without extensive external infrastructure and effectively training themselves.
On X, Dr. Eli David, Co-Founder of cybersecurity firm Deep Instinct, wrote: “Smaller, more efficient models are the way to go for widespread deployment of LLMs.”