Google has poached Anthony Saporito from IBM as Chief Architect to lead development of its "next-generation" CPU. Saporito -- who holds 115 patents -- had a 21-year career at IBM where he was an distinguished engineer and master inventor, working as lead architect for several Z System chips, contributing to several generations of Power and Z System designs with a focus on branch prediction, instruction fetching, and cache design.
The hire comes as Google continues to add muscle to its own semiconductor team, last year hiring Intel veteran Uri Frank as VP of Engineering for server chip design ("Uri brings nearly 25 years of custom CPU design and delivery experience") and others like fellow Intel veteran and System-on-Chip design engineer Alex Gruzman as it builds out teams in California, Israel and India. It has thus-far kept largely quiet on its efforts and approach to building a Google CPU for servers powering cloud workloads, as AWS ploughs ahead with its Arm chips.
Among Saporito's recent successes was the August 2021 launch of IBM's 7nm Telum, its first chip with AI inferencing acceleration. Packing eight CPU cores the Telum chip sits at the heart of IBM's recent z16 mainframe and was the first IBM chip with technology created by the IBM Research AI Hardware Center. He said at launch that the chip -- fabbed on Samsung nodes -- was innovative for its use of an AI accelerator "built right onto the silicon of the chip and we directly connected all of the cores and built an ecosystem up the stack, through the hardware design, through firmware, and through the operating systems, and the software..."
(Some of that chip's innovations were so striking that one observer, Dr Ian Cutress, described them -- tongue-in-cheek but clearly impressed -- as "magic", with ArsTechnica's Jim Salter dubbing them in equal apparent befuddlement as "deeply wierd". Both were referring to the chip's innovative cache design.)
Next-generation Google CPU?
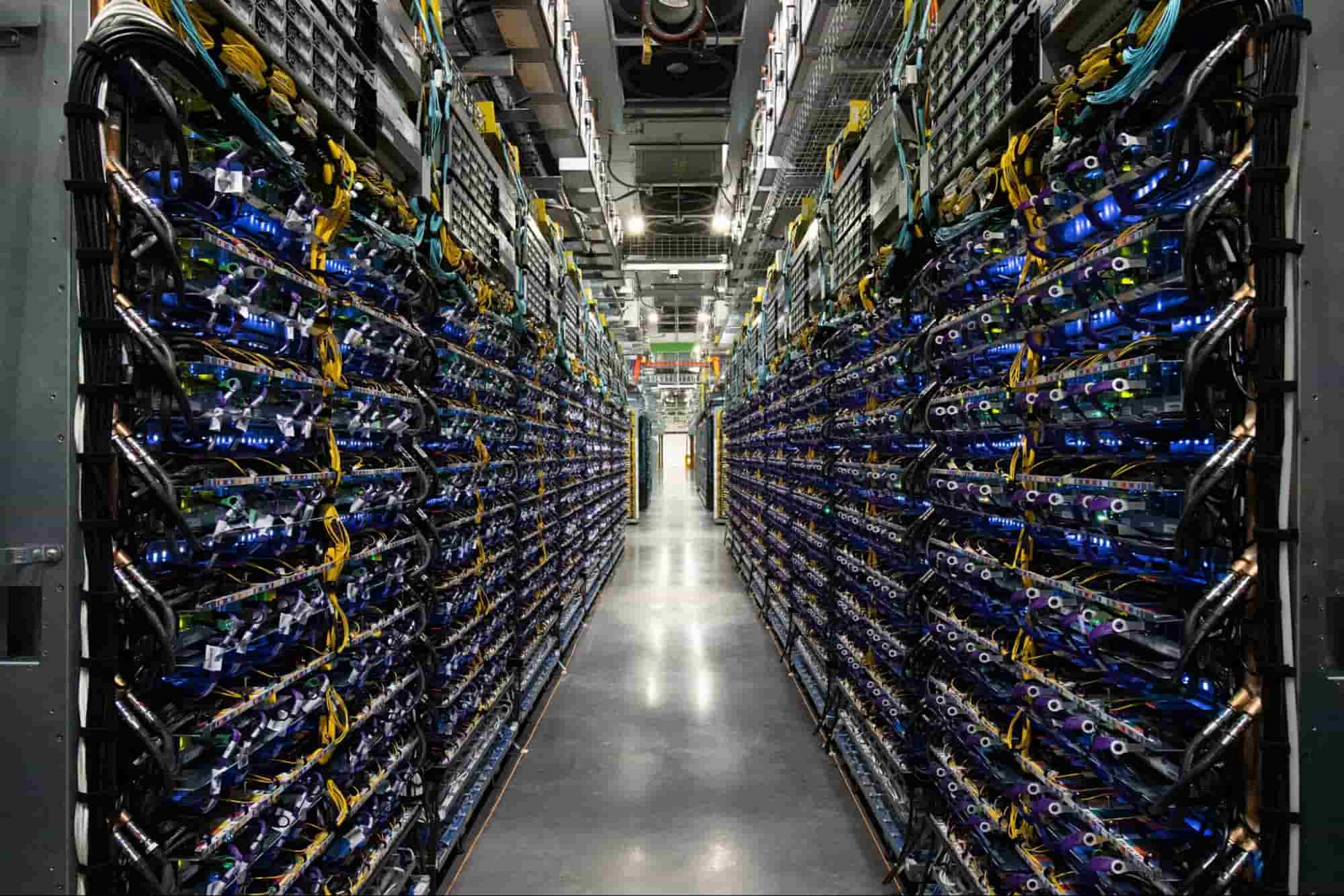
Quite what Saporito means by next-generation Google CPU looks to be something of an open question for the moment. (The Stack has contacted him to ask more but we don't expect an answer as the man barely has his feet under the table...) Google Cloud in particular has made no secret of its plans to ramp up work on custom chips however, with Google Cloud VP of Engineering, Amin Vahdat noting in March 2021: "Custom chips are one way to boost performance and efficiency now that Moore’s Law no longer provides rapid improvements for everyone.
"Compute at Google is at an important inflection point. To date, the motherboard has been our integration point, where we compose CPUs, networking, storage devices, custom accelerators, memory, all from different vendors, into an optimized system. But that’s no longer sufficient: to gain higher performance and to use less power, our workloads demand even deeper integration into the underlying hardware" he blogged at the time.
(In that blog Vahdat emphasised the growing importance of customised System-on-Chip hardware, noting: "Instead of integrating components on a motherboard where they are separated by inches of wires, we are turning to SoC designs where multiple functions sit on the same chip, or on multiple chips inside one package... On an SoC, the latency and bandwidth between different components can be orders of magnitude better, with greatly reduced power and cost compared to composing individual ASICs on a motherboard. Just like on a motherboard, individual functional units (such as CPUs, TPUs, video transcoding, encryption, compression, remote communication, secure data summarization, and more) come from different sources. We buy where it makes sense, build it ourselves where we have to, and aim to build ecosystems that benefit the entire industry.")
Recent social posts by Uri Frank meanwhile suggest that AI inferencing hardware is a priority.
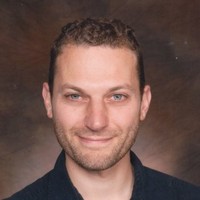
The "build it ourselves" element is clearly a growing focus for Google -- or at least "design it ourselves" for partner fabrication. Creating its own chips is not intrinsically something new for the company. Its "Argos", for example, a class of ASIC known as a Video Coding Unit (VCU) has already replaced large numbers of x86 CPUs in Google data centres. The VCU was created to accelerate video workloads at YouTube, Google Drive, game streaming et al and no doubt across broader enterprise cloud workloads too -- lead software engineer Jeff Calow saying that the company has seen "20-33x improvements in compute efficiency compared to our previous optimized system, which was running software on traditional servers" as a result of its deployment.
(Not without some unexpected hiccups along the way. Calow explained in March 2021: "We deployed a machine in the data center and it failed our burn-in test and one of the chips just didn't come up and we had no idea why. So we’re trying to run a whole bunch of diagnostics and then the hardware tech opened up the carrier machine and noticed that sitting on one of the baffles was this loose screw. And it was basically shorting out one of the voltage regulators and therefore that chip couldn't come up - it was a screw that had come loose in shipping. Nothing caught on fire or anything like that, but it was just like yeah, a screw?")
Anthony Saporito said of his move: "I am extremely excited to share that I have started the next chapter of my career at Google! I am joining an incredibly talented team as the Chief Architect for their next generation CPU. I am both honored and humbled by this incredible opportunity to work alongside industry veterans and future all-stars. 'Hey Google'" he added on LinkedIn, "'let’s deliver some disruptive technology together :)!'"
See also: AI outperforms humans in chip design breakthrough
Google's researchers are also pioneering some intriguing research around semiconductor design. This time last year, a Google team used AI to design physical layouts of computer chips "superior" to those created by humans, in a major breakthrough, as a paper in Nature revealed. The AI-built chip “floorplans” were used to support the design of new Google tensor processing units (TPUs) — or chips deployed to accelerate machine learning workloads.
Using deep reinforcement learning, a research team led by Google’s Azalia Mirhoseini and Anna Goldie generated chip “floorplans” that are “superior or comparable to those produced by humans in all key metrics, including power consumption, performance and chip area”, the June 9, 2021 paper revealed: “Automating and accelerating the chip design process can enable co-design of AI and hardware, yielding high-performance chips customized to important workloads, such as autonomous vehicles, medical devices and data centres,” the research team noted at the time. The method saw superior designs generated in just six hours. The breakthrough is so significant that Andrew Kahng, a world-leading chip expert and professor of computer science at UC San Diego suggested in a companion comment piece in Nature that the innovation may yet keep Moore’s law alive.