Insurance firms are leading the financial services world when it comes to machine learning (ML) strategies.
That’s according to a new survey from the Bank of England (BoE), with 100% of all insurance company respondents saying that they had a clear strategy on ML, compared with 78% of banks, 70% of investment and capital market firms, and 60% of firms in the financial market infrastructure (FMI) and payments category.
The advanced areas when it came to ML deployment were credit underwriting and insurance pricing/underwriting. Other advanced deployments spanned expected loss accounting models; claims handing; monitoring for insider trading or market manipulation; to direct queries within customer interfaces; know your customer (KYC) checks; trading strategy and execution; payments authorisation; and staff wellness programmes.
The BoE machine learning survey found that developing in-house capabilities had clearly been a priority for most, with 83% of respondents developing and implementing ML applications internally at their firm.
A key takeway: "Cross-functional, multi-disciplinary teams tend to be the most effective at developing and deploying ML applications that are safe and responsible" respondents told the Bank of England.
(The central bank wrote to 168 firms, with 71 submitting responses. The report follows a similar 2019 survey and the establishment of the Artificial Intelligence Public-Private Forum (AIPPF) in 2020, which explored various barriers to adoption and challenges related to the use of AI)/ML, as well as ways to address such barriers.)
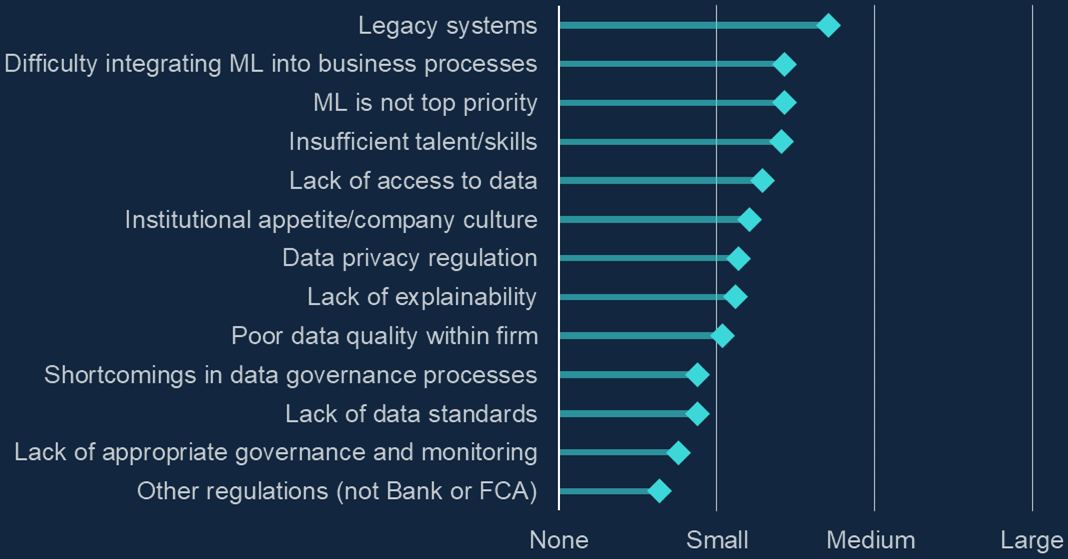
Across organisations that are often rife with complex legacy technology systems and codebases, the biggest hurdle to broader ML adoption was perhaps unsurprising -- "legacy systems and associated technology infrastructure" -- and likely also captures the extent to which these heterogeneous, often siloed systems are also home to critical data that cannot always be pulled out easily into a datalake/used to run training on.
As the 2022 Bank of England machine learning report says: "ML applications and the processes around them often need up-to-date hardware, database infrastructure, and operating systems to run effectively and efficiently. That is why developing, deploying and/or integrating ML with legacy systems can become challenging."
(Those setting out on this journey could do worse that to listen to Allianz CDO Sudaman Mohanchandralal, who built a data strategy from "nothing" and now uses 20 TB of structured data and 2PB of unstructured data to inform product pricing and other enterprise decisions. He earlier told The Stack that his guidance for others early on such journeys was "please don’t kickstart data culture initiatives or heavy data investments in terms of infrastructure until you have a serious business case — and do it in a short loop, maximizing value. We go to the board every year to ask for funding by showing what we have brought in, as revenue, for the company, so there is an attribution logic to the Data Office activities. I can proudly say ‘this is the money which our initiatives have brought in; this is the operational process. This is the growth that we have been able to bring.’")
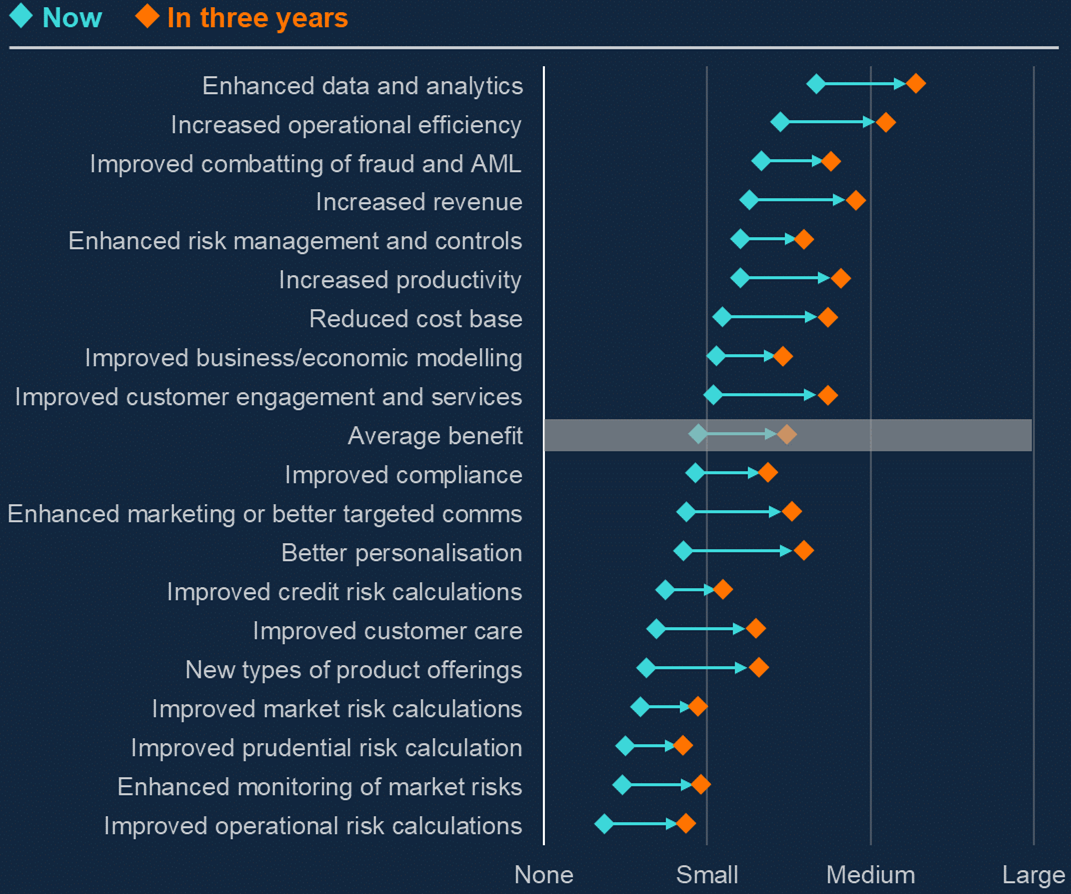
When it came to types of machine learning, the most commonly used methods are tree-based models, regression (excluding simple linear regression), and neural networks. Models in the "other" category included Bayesian approaches, alternating least squares, grid search techniques, and image recognition techniques.
("Some firms were unsure about the underlying model because it was created and developed by a third-party provider" the Bank of England noted in its report, which was published on October 11, 2022.)
"Firms often use tree-based ensemble methods, such as ‘random forests’ and ‘XGBoost’, which consist of a multitude of – often large – decision trees whose individual predictions are averaged" the BoE noted.
"Respondents said these methods have been relatively successful for prediction in traditional financial data analysis contexts. Neural networks tend to be used to make forecasts based on historical information and find complex relations between non-linear variables. Respondents also used some ML regression techniques, such as logistic and penalised regression, for forecasting and time series modelling. Most respondents’ ML applications used, on average, a combination of two methods. In one use case, a firm used nine separate ML techniques in a single application."
A number of respondents cited regulatory uncertainty around ML deployments as a hindrance. The Bank directed them to a discussion paper (DP) it jointly published with the PRA and FCA on how the current UK regulatory framework applies to AI/ML. The DP also explores how policy can best support further safe and responsible AI/ML adoption and whether additional clarification of the existing regulatory framework may be helpful.
"Readers of this survey are encouraged to engage with the DP and submit a response."