The developers of Generative AI (GenAI) models are failing to adequately communicate the limitations and unreliability of their creations, the US Government Audit Office (GAO) has warned.
In a report released yesterday, investigators warned that vendors' focus on the whizzbang capabilities of their models comes at the expense of honesty about the true problems with GenAI.
The GAO probe considered the "training, development and deployment considerations", setting out a range of major issues with GenAI and warning that the public has been left in the dark about key aspects of the training of models as well as their propensity for hallucinations and "confabulations".
"Commercial developers face some limitations in responsibly developing and deploying generative AI technologies to ensure that they are safe and trustworthy," GAO wrote. "Developers recognize that their models are not fully reliable, and that user judgment should play a role in accepting model outputs.
"However, they may not advertise these limitations and instead focus on capabilities and improvements to models when new iterations are released.
"Furthermore, generative AI models may be more reliable for some applications over others and a user may use a model in a context where it may be particularly unreliable."
Why you can't trust an LLM
Officials pointed out that GenAI models are prone to spewing incorrect or biased outputs - a finding that will be familiar to anyone who's beheld low-grade marketing materials or other generic content written by a large language model. These problems persist "despite mitigation efforts" by their creators.
"[Models] can produce 'confabulations' and 'hallucinations' - confidently stated but erroneous content that may mislead or deceive users," GAO continued. "Such unintended outputs may have significant consequences, such as the generation and publication of explicit images of an unwilling subject or instructions on how to create weapons."
Additionally, models are insecure because malicious users are "constantly looking for methods to circumvent model safeguards" using basic techniques which "do not require advanced programming knowledge or technical savvy".
"Rather, attackers may only need to rely on the ability to craft text prompts to achieve their goals," GAO wrote. "Commercial developers are aware of these realities and the limitations they impose on the responsible deployment of AI models."
READ MORE: What 'went wrong' with GenAI in business and the enterprise? Princeton professor warns of 'unsolved challenges'
Generating novel attack paths
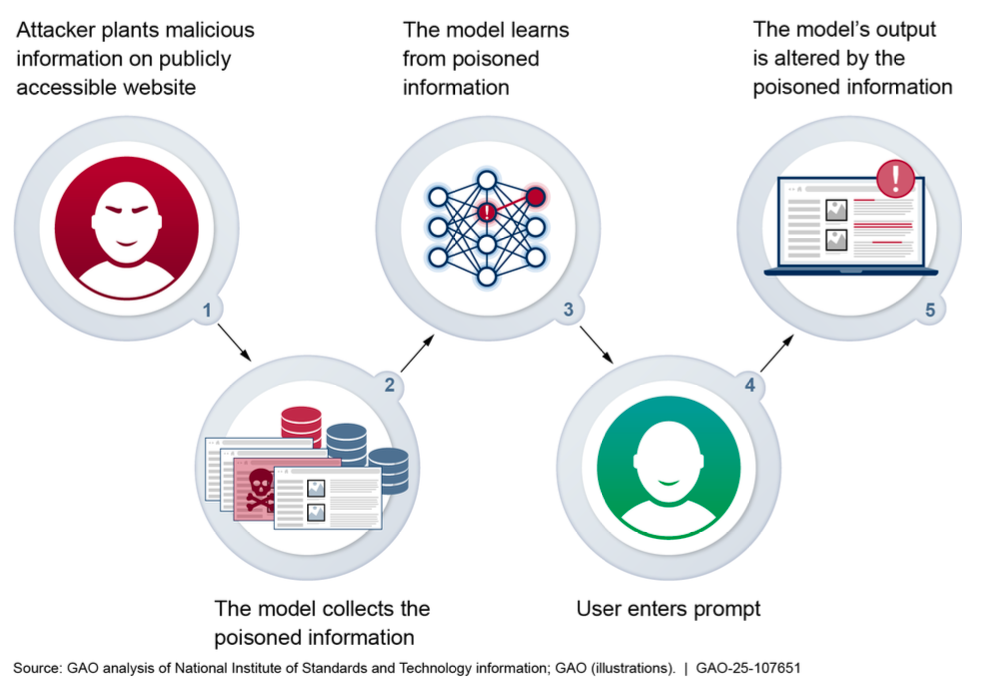
The watchdog discussed data poisoning attacks (illustrated in the image above) as an example of an emerging security threat generated by the rise of AI. These attacks change the behaviour and outputs of a generative AI system by manipulating its training data or process.
"Foundation models are especially susceptible to poisoning attacks when training data are scraped from public sources," the watchdog warned. "In a data poisoning attack, an adversary controls a subset of the training data by either inserting or modifying training samples. Executing data poisoning can be as simple as purchasing a small fraction of expired domains from known data sources."
Developers were also accused of failing to be totally clear about the sources of the data used to train models, which ranges from information scraped from the internet to copyrighted materials or social media posts.
Some are quietly collecting data from their own users, including prompt inputs, account details, IP address, location and details or their interaction with the service and other applications.
However, information about the specifics of training datasets is "not entirely available to the public", GAO said. One expert told investigators that "transparency of training data for generative AI models has worsened over time".
"The commercial developers we met with did not disclose detailed information about their training datasets beyond high-level information identified in model cards and other relevant documentation," GAO added. "For example, many stated that their training data consist of information publicly available on the internet.
"However, without access to detailed information about the processes by which they curate their data to abide with internal trust, privacy, and safety policies, we cannot evaluate the efficacy of those processes. According to documentation that describe their models, developers did not share these processes and maintain that their models’ training data are proprietary."
Developers have also failed to disclose how much of their training data includes copyrighted information, with some arguing that using materials owned by someone else is protected as fair use.
Training datasets may also contain private and personal information, with removal and redaction far from guaranteed.
"For example, it may be relatively easy to find and remove an e-mail address as compared to an identification number," the paper advised.