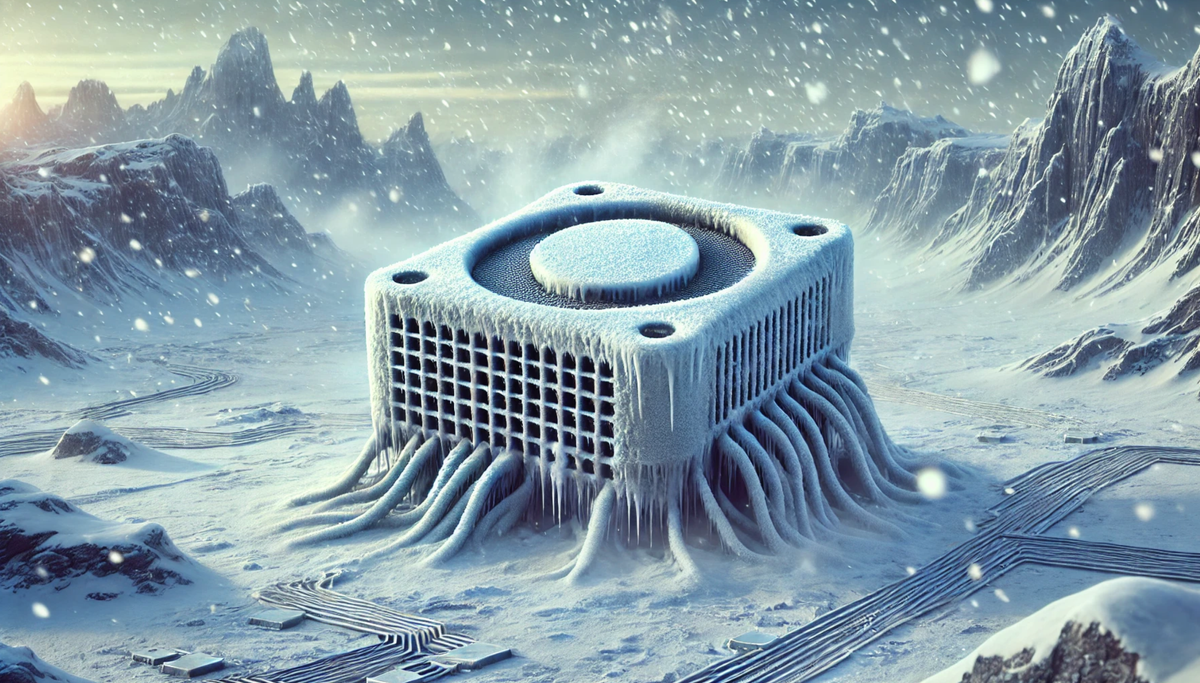
It's been just two years since the dawn of ChatGPT. Now "diminishing returns" from recent GenAI upgrades have sparked fears that the AI boom is fizzling out and that the path to AI superintelligence may not be quite as straight and obvious as it once seemed.
That’s the view of one ever-reliable AI doomsayer in the wake of a report suggesting that the rate of GPT improvements was slowing. But are we really about to exit the age of AI, before it has even begun? To find out, The Stack spoke to experts on what the future might hold.
Professor Gary Marcus, a known Large Language Model sceptic hailed reports of slowing GenAI progress as proof that LLM scaling was at an end, saying: "The economics will likely never make sense: additional training is expensive, the more scaling, the more costly."
He added: “The economics are likely to be grim. Sky high valuation of companies like OpenAI and Microsoft are largely based on the notion that LLMs will, with continued scaling, become artificial general intelligence. As I have always warned, that’s just a fantasy.”
Marcus’s perspective is just one gloomy voice - Goldman Sachs has predicted that a trillion dollars will need to be spent on grid upgrades, chips and data infrastructure to power the continued growth of generative AI.
It’s worth noting, however, that Amir Efrati of The Information, author of the original report, responded by saying, “The future seems to be LLMs combined with reasoning models that do better with more inference power. The sky isn’t falling.”
READ MORE: Microsoft study shows that GenAI struggles with one simple task
The rocky path to AGI
In a November 2023 speech at Cambridge University, OpenAI’s Sam Altman already made it clear that he believes that simply scaling LLMs will not be sufficient to achieve ‘Artificial General Intelligence’.He said, ‘"I think we need another breakthrough.”
The diminishing returns seen from LLMs do not mark a ‘wall’ that prevents further AI progress, says Fergus Dyer-Smith, CEO and founder of AI and video production company Wooshii.
The real opportunity does not lie in simply making models more powerful, Dyer-Smith argues.
He says: “The real opportunity lies in the application layer, leveraging these foundation models and their sophisticated reasoning layers. The addressable market isn’t the $350B software industry - it’s the multi-trillion dollar services sector. For B2B, transforming traditional professional services into scalable AI-powered solutions is a huge opportunity.
“The future likely belongs to companies that have managed to effectively harness these models and benefit as they improve rather than be wiped out as they replace their core function.”
“While there is evidence that traditional LLM scaling is hitting a wall of diminishing returns, it would be premature to declare the end of the AI boom. What we are seeing isn’t a wall - it’s a pivot point."
READ MORE: Elon Musk to double power of world's largest AI supercomputer
The cost of GenAI progress
The sheer expense of training large foundation models is already driving the market away from large models towards smaller ones, says Erik Schwartz, Chief AI Officer of IT consulting firm Tricon Infotech.
He told The Stack: “Each new release of a large model seems to have only marginal gains over previous versions, which indicates that the providers are seeing diminishing returns. For example, while GPT-4 shows improvements over GPT-3.5 in reasoning and specialised tasks, the gains aren't proportional to the massive increase in computational resources required."
Instead of simply throwing more compute at models, pioneers in the AI market are shifting towards new approaches, Schwartz explains.
“There is a trend that we are seeing in that marketplace towards smaller models as the large foundation models are becoming quite expensive to build, train, and iterate on. The larger models are in the 400-450B+ parameter size, where the smaller models are in the 70B down to 7-8B parameters," he adds.
Other approaches are already yielding results, Schwarz says - including integrating AI agents into workflows.
"There is a trend towards new architectures to help address some of these constraints, including the ability to learn, think, and reason more deeply," he says. "Agentic workflows are also allowing models to create more specialisation or act as subject matter experts for a particular domain or set of tools.
“For instance, specialised AI agents can now handle complex financial analysis or medical diagnosis with domain-specific knowledge and tool integration, delivering more precise and reliable results than general-purpose AI."
Schwarz does not believe foundational AI companies such as OpenAI are over-valued, but that companies that are "wrappers" around larger models are at risk.
He continues: "New features will be launched by major models that will wipe out entire classes of application, but this consolidation is expected, much like we saw desktop applications that were subsumed by operating system features in Windows or MacOS."
Schwarz believes that there is still "quite a bit of growth" left in the AI market, and that a new "maturity model" will continue to expand the tech’s impact.
He says: “Today, we are seeing AI used for personal productivity use cases, such as email composition, meeting summarisation, and code assistance. Soon, we will see existing business models and workflows start to incorporate AI to remove friction and further smooth out very manual and fragile processes - think intelligent document processing in legal firms or automated underwriting in insurance. Looking further ahead, a new set of AI-native companies will emerge, creating entirely new offerings we didn't even know we needed.”
READ MORE: Are hallucinating GenAI models careless or just plain ignorant? Google researchers found out
In the bleak AI midwinter
For decades, AI "winters" in which progress slows and innovation stagnates have been part of the evolution of the technology, but there are already signs that the field is moving beyond the limits of GPT-style transformers, Dr Andrew Bolster, senior R&D manager for data science at cybersecurity firm Black Duck tells us.
Bolster says: “We may indeed be at some kind of ‘dead end’ with the utility of GPT-style transformer architectures, but we’re certainly not at the ‘End of AI’.”
The current AI research field illustrates that there are new ideas out there, potentially showing new ways forward for the field, Bolster says.
Bolster says: “We can see an increasing emphasis on reducing computational complexity of training, the difference between ‘data’ and ‘experience’, tighter control of ‘how to forget’, and more secure AI stacks.
“The field overall is maturing beyond the explosive growth in the number of parameters and the trillions of training tokens used, towards a (hopefully) more mature and thoughtful approach to how to develop these technologies."