Futurologist Roy Amara once said, “We tend to overestimate the effect of a technology in the short run and underestimate the effect in the long run.” Similarly, Bill Gates stated, “People often overestimate what will happen in the next two years and underestimate what will happen in ten.” Based on these thoughts, what can we predict around the impact of AI?
The first consideration is that making any concrete predictions is hard. We expected full self-driving cars to enter the market a while back, but the path to full autonomy will be a long one. McKinsey estimates that only four percent of cars sold will have full self-driving by 2030. The UK Government has set aside £100 million of funding to get involved in this sector, betting on 38,000 new jobs and a new industry worth £42 billion, but this is by no means certain. At the same time, we have seen the likes of ChatGPT and MidJourney spring up around chat and image generation over the past few months. To a casual observer, these may look like much harder problems to solve, but they are seeing faster innovation and new launches almost daily.
Underneath all these projects is the software to translate what we see and use into a form that computers can understand, and then provide information or other results back to us. These projects are based on code and data. How that code and that data are managed will be critical to future successes.
The role for open source
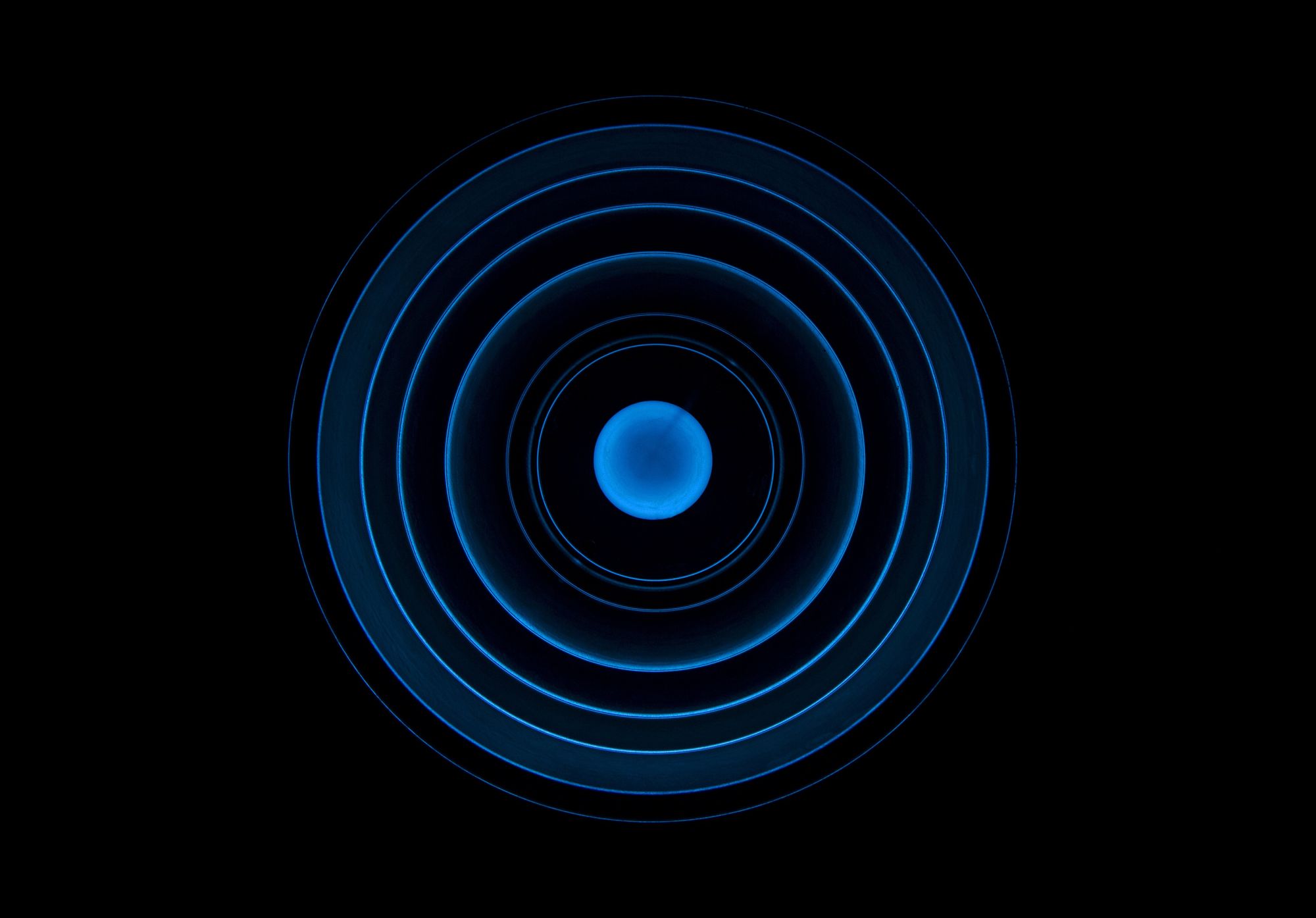
For software, open source has been essential to the success of multiple markets. The launch of WordPress twenty years ago made it easier, faster, and cheaper to build websites and sped up the development of the whole sector around the Internet. Similarly, open source databases made it easier and faster to build applications and store data over time, supporting the development of online services that operate at scales we could not have imagined. According to research by OpenUK, 90 percent of companies use some form of open source software.
For AI, open source will have a role to play in bringing this technology to mass adoption. We are already seeing large amounts of AI software being released under open source licenses. However, these releases are not super useful to the market without the large language models (LLMs) that are applied to sets of data. These models are often proprietary, but Meta has now launched its Llama 2 LLM under a license that makes it possible to use for commercial purposes. It is not open source, but it is more open than a lot of other options are.
Training your own model requires a large amount of data, which is also often proprietary, and huge volumes of computing power, which is a significant challenge. As examples, it’s estimated that GPT-3 from OpenAI cost more than $12million to train and more than 350GB of memory, while GPT-4’s training costs are estimated at more than $100million. The best solutions on the market such as ChatGPT also rely on a lot of human labour to assist with training and annotation for data and accuracy, which is itself costly. Any advances around LLMs will be based on taking existing LLMs and developing them further. For this to happen, we have to have permissions in place.
In the realm of software, this evolution took place using software licenses that allowed sharing and re-use based on the definitions created by the Open Source Initiative. These licenses were developed for code first and foremost, so for other areas like media, Creative Commons is used instead.
See also: AI has simulated the earth’s surface to a whole new level of detail
With AI, which covers the software, models and data used to create results, we will see both open source licenses applied to the code as well as open source and other, possibly specially created, licenses to be applied to the AI training data, model, and output. These licenses will manage how people are able to use resources and for what purposes. For any new licenses to be considered as “open source” they will have to be available for use without commercial or use restrictions, in line with other licenses certified by the OSI.
In the end, I think we will get something like Wikipedia in the AI space, where we not only have open source software but all the other components too, and where the community can then use the results where they are available. Alongside this, we could look to establish an official and consistent set of terminology for code, data, weights, and other requirements around AI. This could be comparable to how the US Department of Agriculture has specific certification for items that are labelled as Organic. Similar to how you can’t just call something Organic because in your definition it means being made from Organic Chemistry compounds, there is probably a need and an opportunity for “OSI Open Source”, “OSI Ethical Weights” and similar labels and certification processes.
What is the longer-term impact likely to be?
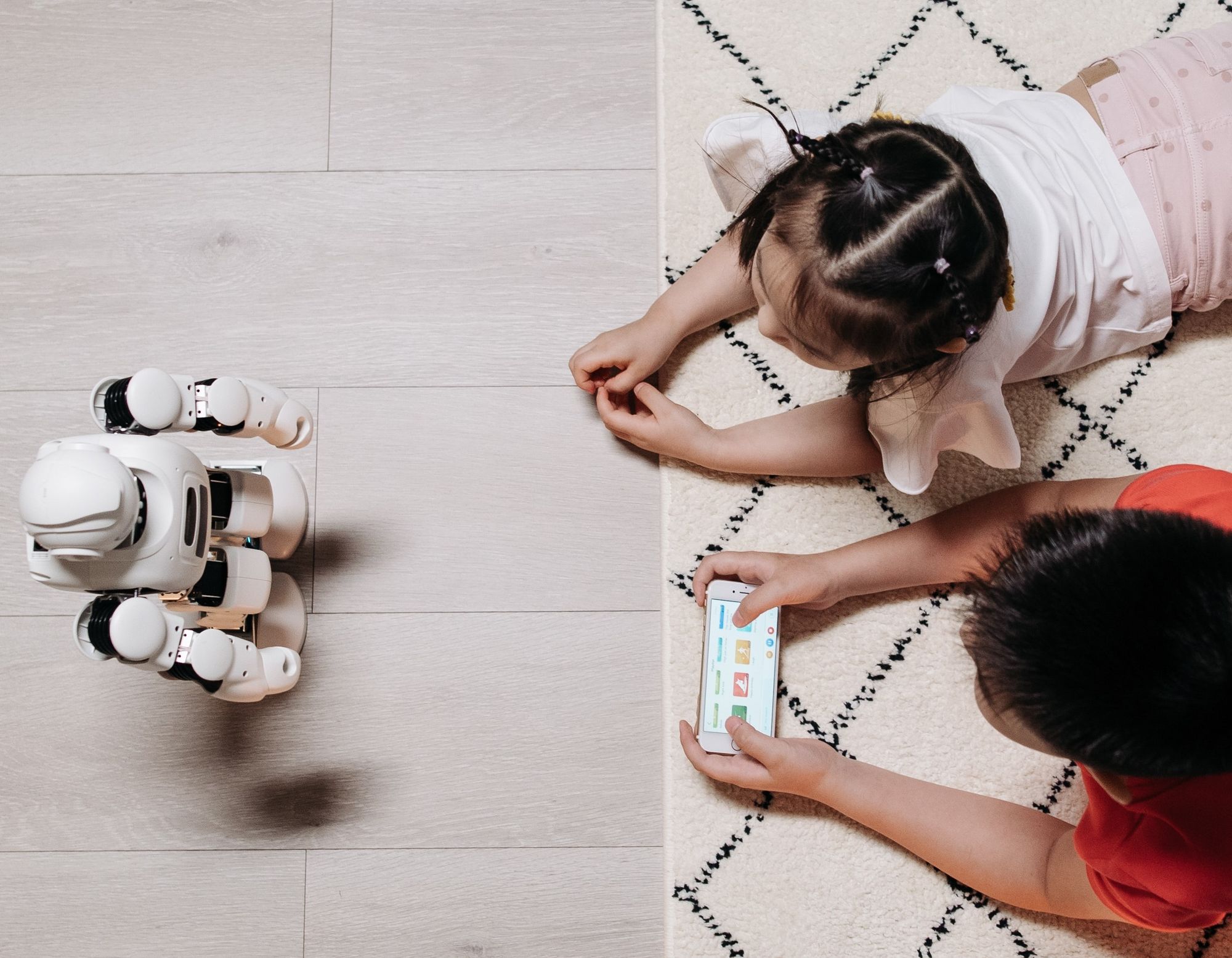
Alongside creating and managing the components that make up AI systems, we also have to look at the impact that those AI services will have over time. Goldman Sachs Research published that AI may displace the equivalent of up to 300 million jobs, while more than 900 million will see some form of automation using AI. Similarly, PwC estimated that AI could deliver a boost in global GDP of around $15trillion by 2030 but that 30 percent of jobs would be affected.
However, all this talk of replacing humans with AI seems to forget that many business problems are, at heart, people problems. These kinds of issues are hard to solve using machines, no matter how smart they are, because they have a lot more nuances to them, and they tend to have longer-term consequences that modelling will not take into account. This ability to think through issues and look at both short-term and longer-term impacts is not something that is currently well-documented in AI. It’s hard for people to think strategically and take all the factors into account, so it is difficult for AI to do the same around unintended consequences.
There is also the aspect of motivation to bear in mind. While we might want to apply AI to do things for us, many of the tasks will be around supporting decisions that people make or actions they carry out. At this point, human interaction can be more appropriate than AI apps that are limited in how they can interact with users. Think about fitness apps for example - while an app can develop an awesome program for you and track your progress, it is no match for a personal trainer or group of peers when it comes to encouragement and understanding what might be needed to help you carry out tasks and get your results. While some people respond well to gamification and apps, others do not.
On top of this, reasoning is the great challenge for using AI for important decisions. Generally, we as humans want to validate the decisions we can make, we want to understand what facts or data were considered to come up with those options, what alternatives were evaluated and which was selected and why. This functionality is currently lacking, at least in mainstream LLMs. Without that ability to trace back and understand why we are getting the recommendations that are coming through, we won’t be able to trust that our AI services will be reliable over time, or that we will be able to understand why something did not go according to plan.
I consider myself an “AI realist” - while AI has huge potential, it is not as smart as many make it out to be. It gives the appearance of being clever because we can’t see the workings and all the effort that goes into the results we see appear based on our prompts or in our application requests. Looking ahead, AI will produce some fantastic results around consumer services, but will these live up to the hype that is currently being created? At the same time, the long-term impact will go further than we expect.
What will the end result be around AI? It is impossible to predict the future with any accuracy, but the results should go further than we are currently able to see, so we should not underestimate the work going in. To make this process equitable and understandable, we have to keep the vision of open source in mind so that everyone in the community can take advantage of these developments, rather than keeping them in the hands of the few.